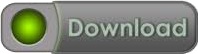

This means that we need to know whether our explained variation (65%) is higher than would be variation explained by some random variable. Even if the explanatory variable is a randomly generated value, it will explain the non-zero amount of variation (you can try it to see!). Some details below:īefore we start to interpret the results, we often want to make sure that there is something to interpret. These are usually numbers you need to include when you are reporting the results of the regression. We can apply generic function summary on the object which stores the linear model results, to get further numbers:Ĭommented output reports the most important variables: apart to the regression coefficients also the coefficient of determination (r 2), and the value of F statistic and P-value. We can argue that the intercept coefficient does not make practical sense (at zero speed, the braking distance is obviously zero, not -17.6 feet), but let's ignore this annoying detail now and let's focus on other statistical details. Using these coefficients, we can create equation to calculate fitted values: The function 'lm' returns estimated regression coefficients, namely intercept and slope of the regression. Lm_cars <- lm ( dist ~ speed, data = dist ) To calculate linear regression model, we use the function lm (linear model), which uses the same formula interface as the plot function: We can draw the relationship, using the plot function with variables defined using formula interface: dependent_variable ~ independent_variable, data = ame:
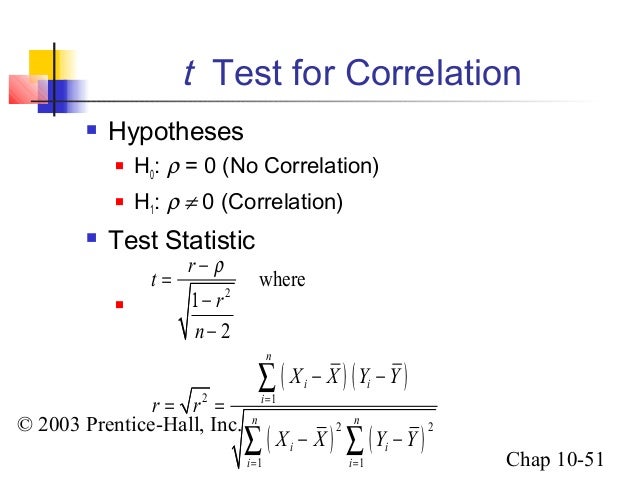
Obviously, dist is dependent variable and speed is independent (increasing speed is likely increasing the breaking distance, not the other way around).
#Calculator f statistic multiple regression driver#
In this example, let's 1) calculate linear regression using example data and fit the regression equation, 2) predict fitted values, 3) calculate explained variation (coefficient of determination, r 2), and test the statistical significance, and 4) plot the regression line onto the scatterplot.ĭata we will use here are in the dataset cars, with two variables, dist (the distance the car is driving after driver pressed the break) and speed (the speed of the car at the moment when the break was pressed). In reality, we are going to let Minitab calculate the F* statistic and the P-value for us.Linear regression is a common statistical method to quantify the relationship of two quantitative variables, where one can be considered as dependent on the other.

The P-value is determined by comparing F* to an F distribution with 1 numerator degree of freedom and n-2 denominator degrees of freedom. \(MSE=\dfrac\).Īs always, the P-value is obtained by answering the question: "What is the probability that we’d get an F* statistic as large as we did, if the null hypothesis is true?" We already know the " mean square error ( MSE)" is defined as: Let's tackle a few more columns of the analysis of variance table, namely the " mean square" column, labled MS, and the F-statistic column, labeled F. The sums of squares add up: SSTO = SSR + SSE.And the degrees of freedom add up: 1 + 47 = 48. The degrees of freedom associated with SSE is n-2 = 49-2 = 47. The degrees of freedom associated with SSTO is n-1 = 49-1 = 48. The degrees of freedom associated with SSR will always be 1 for the simple linear regression model.Recall that there were 49 states in the data set.
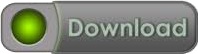